Detecting Patterns and Defect Anomalies in Physical Identity Data with AI
In an increasingly digital world, the management and analysis of physical identity and access data have become crucial for security, fraud detection, audits, compliance and personalized services. Analyzing this data to detect patterns and anomalies is essential for ensuring accuracy, preventing identity theft, and improving system efficiency. Here’s how AI techniques are making this possible.
Pattern Recognition with Machine Learning
AI’s ability to recognize patterns in physical identity data hinges on machine learning (ML) algorithms. These algorithms are trained on large datasets to identify consistent traits or features in physical Identity data.
Anomaly Detection with Deep Learning
Anomalies in identity data—such as attempts at identity fraud, identity or card duplication, unauthorized access etc. —are critical to detect in real time. Deep learning techniques, particularly autoencoders and recurrent neural networks (RNNs), excel at this task. Any significant deviation from identity or access card patterns over time can be flagged by the model automatically.
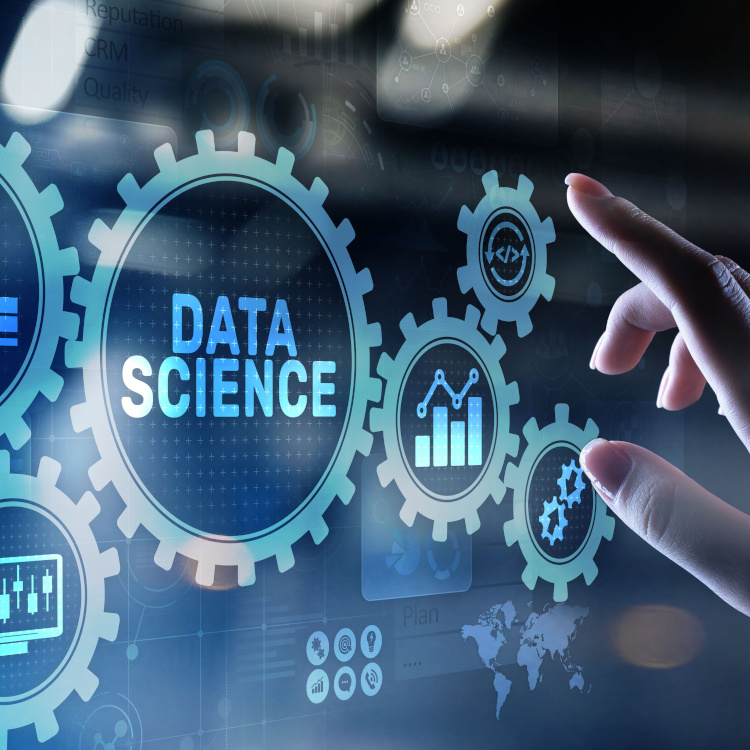
AI-Powered Clustering for Identity Management
In the context of physical identity management, clustering helps in organizing and categorizing vast datasets, making it easier to identify unusual patterns or outliers. For instance, if a cluster of data points deviates significantly from established patterns, it may indicate an attempt to bypass security systems.
AI systems can continuously monitor identity data and adapt to new patterns over time. This is crucial as fraud tactics evolve. By using adaptive learning techniques, AI models can update their parameters based on new data, ensuring that they remain effective against emerging threats. As identity and physical access verification becomes more critical in our digital age, these AI-driven methods will continue to enhance security and streamline PIAM functions across various sectors and domains.